The place for bias in the future of accounting
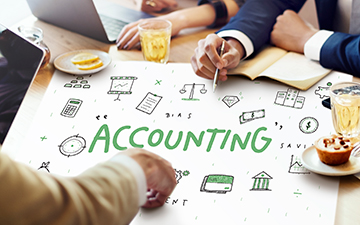
Banishing machine bias requires human self-awareness
VANCOUVER – Let’s face it — as humans, we’re biased. We tend to trust and gravitate toward those who resemble ourselves. Conversely, we mistrust “strangers” who look and sound different from our own norm. We interpret situations based on familiar contexts and overlook other perspectives. Even though we see ourselves as tolerant and openminded, our natural instinct is to be protective.
As chartered professional accountants, our code of professional conduct requires that we be objective — that is, that we not let our professional and business decisions be swayed by bias, conflicts of interest or undue influence. Overcoming bias is therefore both a professional obligation and a personal pursuit to avoid sub-par decisions.
For our ancestors, bias was a necessity. In previous eras, other cultures posed a real threat; being mistrustful was often a matter of survival. In the modern world, thankfully, this has changed. While it would be naïve to say that the world is one big happy community, we’ve at least evolved to the point where we recognize that diversity greatly enriches our lives rather than threatening us.
![]() |
Laura Friedrich and Brian Friedrich are the principals of friedrich & friedrich corporation. |
Despite our progress, however, it's unrealistic to think that we can simply drop our tendency for bias that was arguably adaptive for so long. Moreover, we still — and will always — rely on unconscious bias to help us make decisions. Biases act as mental shortcuts. Removing them altogether would mean that we’d risk being overwhelmed by having to consciously evaluate every factor of a decision rather than unconsciously give weight to some elements we already “know.”
So bias is not always a four-letter word. Rather than seeing it as something to be ashamed of and hidden, society would be much better off if we recognize bias for what it is: a natural human trait. By removing the stigma of bias, we can work collectively to move past it. We need to individually recognize that each of us has inherent biases that are influenced by our background, culture and environment. By increasing the diversity of the experiences we seek out, we can teach ourselves the limitations and falsehoods associated with the biases that we once held, and help others do the same.
The need to examine and overcome unhelpful biases has never been more acute. As machine learning and artificial intelligence become increasingly common in business, it is incumbent upon us as trusted professionals to ensure that human biases aren’t perpetuated in the data sets and algorithms at the core of systems.
We have a propensity to have confidence in computer-generated results once we’ve accepted a tool. How many times have you followed GPS guidance that seemed faulty in hindsight? Or automatically relied on a flawed Excel spreadsheet without re-checking its formulas and logic? As systems become even more autonomous, the risk of over-reliance becomes even greater. If we’re not careful, biases will be entrenched irrevocably into the intelligent systems on which we will come to rely for decision-making.
A key requirement in overcoming bias in AI lies in ensuring that companies invest sufficient funds into collecting data that is truly representative and reflective of the relevant population and circumstances, rather than settling for historical data that reflects inequalities and flawed reasoning. If computers are to help us make better decisions, we need to recognize that the decisions we made in the past were not always the best ones. When developing algorithms, companies need to proactively assess the inputs and processing of algorithms to maximize impartiality, and also evaluate the outputs for reasonableness.
AI-enhanced decision support systems in particular need to be critically reviewed by teams with diverse backgrounds and skill sets — including professional accountants — to uncover machine-learned biases as they develop. Review teams with varied motivations or incentives should also be chosen so that any self-interest aspects are rooted out and examined. Being able to detect bias in machines, however, presumes our ability to detect biases in our own thoughts and decision-making processes, requiring self-awareness and practice.
Which brings the spotlight back to the humans in the room. As we move forward in the digital age there is a distinct place for bias — right out front in the spotlight where we can see it and address it appropriately.
Brian Friedrich, MEd, LL.M, C.Dir, FCPA, FCGA and Laura Friedrich, MSc, CIA, FCPA, FCGA are the principals of friedrich & friedrich corporation. For over 20 years, the firm has built institutional capacity, implemented competency-based education, and developed strategic, program, governance and ethics guidance for established and emerging professional and regulatory organizations. Laura and Brian facilitate seminars and workshops in these domains both in-person and through the mobile learning application ProDio.
(0) Comments